As the field of biology continues to evolve, the integration of artificial intelligence (AI) has revolutionized scientific research by unlocking hidden secrets within cells.
The use of AI models has allowed researchers to analyze vast amounts of raw cellular data with unprecedented speed and accuracy, leading to groundbreaking discoveries that were previously out of reach for traditional methods.
In this article, we will delve into the latest breakthroughs in biology research facilitated by AI, including the creation of elephant stem cells, the discovery of a mysterious galaxy, and the identification of rare kidney cells through foundation models.
How machine learning could help biology?
In recent years, the intersection of artificial intelligence (AI) and cellular biology has led to groundbreaking discoveries and transformative advancements. Researchers are harnessing the power of machine learning algorithms to unravel the mysteries of cells, proteins, and biological processes. Let’s delve into how AI is shaping the future of cellular biology.
1. Protein structure prediction
One of the most significant breakthroughs facilitated by AI is in predicting the D structures of proteins. Traditionally, determining protein folding was a labor-intensive process, often taking months or even years. However, AI tools like AlphaFold, developed by DeepMind, have revolutionized this field. AlphaFold leverages machine learning to predict protein structures accurately and rapidly. By analyzing DNA sequences and evolutionary histories, AlphaFold can generate D models for thousands of proteins. Researchers can now explore protein functions, interactions, and potential drug targets more efficiently.
In a recent study published in Nature, researchers compared the efficiency of AlphaFold to that of a single human researcher in predicting the 3D structures of proteins. The results showed that AlphaFold was not only faster but also more accurate than a human scientist.
The study involved 147 challenging protein targets, which were selected based on their difficulty level and lack of structural information. Researchers asked 21 expert biochemists to predict the structure of these proteins using conventional methods, while AlphaFold was also given the same challenge. The results showed that AlphaFold outperformed humans in terms of accuracy, with a median global distance test score (GDT) of 90, compared to a GDT of 81 for human scientists.
Moreover, AlphaFold was able to predict structures much faster than humans, taking only a few days to solve each protein, while humans took several weeks or months. This speed and accuracy have the potential to transform the field of biology by enabling researchers to quickly and accurately identify new drug targets and understand the mechanisms behind diseases at a molecular level.
2. Drug discovery and design
AI plays a pivotal role in drug discovery. By analyzing vast datasets, AI algorithms identify potential drug candidates and predict their interactions with cellular components. These predictions guide researchers in designing novel drugs with enhanced efficacy and reduced side effects. Machine learning models can also optimize drug properties, such as bioavailability and binding affinity, leading to faster drug development cycles.
Several groundbreaking projects and companies are pushing the boundaries of AI-driven drug discovery:
- Atomwise: Their platform, AtomNet, uses deep learning techniques to predict the binding of small molecules to proteins, enabling rapid screening of potential drug candidates.
- Exscientia: A pioneer in AI-driven drug design, this company has successfully brought the first AI-designed drug into clinical trials for the treatment of obsessive-compulsive disorder.
- BenevolentAI: Their Knowledge Graph platform combines massive biomedical data with AI to identify novel drug targets and repurpose existing drugs for new diseases.
- Insilico Medicine: This company uses generative AI models to design new molecules with desired properties, accelerating the discovery of drugs for age-related diseases.
AI promises to make drug discovery faster, cheaper, and more successful. While still in its relatively early stages, the potential of AI to address complex diseases, improve target selectivity, and reduce toxicity in drug development is undeniable. As AI algorithms continue to improve and datasets become more comprehensive, we can expect even more revolutionary breakthroughs in drug discovery, leading to a new era of effective and personalized therapies.
3. Biomarker identification
Biomarkers, as biological indicators of diseases, are fundamental to modern medicine. They are essential for early diagnosis, risk assessment, disease monitoring, and designing personalized treatments. Traditionally, the search for reliable biomarkers has been a complex and time-consuming process. However, the integration of artificial intelligence (AI) is transforming this field, driving faster and more accurate biomarker discovery.
AI algorithms analyze genomic, proteomic, and metabolomic data to identify relevant biomarkers. These markers aid in early disease detection, personalized medicine, and monitoring treatment effectiveness. For instance, AI-driven analyses have identified specific gene expression patterns associated with cancer subtypes, allowing for targeted therapies.
AI-driven biomarker identification offers also others numerous benefits:
It facilitates faster discovery of biomarkers, potentially leading to earlier disease diagnosis and more rapid development of new treatments. AI also contributes to improved accuracy in biomarker identification, a critical step towards precise diagnostics and prognostics. Moreover, AI paves the way for personalized medicine by helping identify biomarkers that predict how patients will respond to specific therapies. The use of AI algorithms can uncover previously unknown biomarkers, providing novel insights and ultimately leading to the development of targeted therapies
4. Cellular image analysis
Artificial intelligence is revolutionizing how we analyze microscopic images of cells. AI algorithms, particularly deep learning techniques, can identify and outline individual cells within complex images, measure and quantify their size, shape, texture, and other visual features, track cell movement over time, and even categorize cells into different types or states. This offers several advantages over traditional analysis methods. AI can process huge amounts of image data much faster than humans, reducing bias and providing more accurate, consistent results. It can also detect subtle patterns and changes in cells that might be overlooked by human experts, potentially leading to novel discoveries.
The applications of AI in cellular image analysis are rapidly expanding. In pathology, AI assists in cancer diagnosis by identifying abnormal cells and tissues. Pharmaceutical companies use AI to accelerate drug development by analyzing cellular responses to potential treatments. Meanwhile, researchers in cell biology, immunology, and other fields gain deeper insights into cell structure, function, and behavior with the help of AI analysis tools.
While incredibly powerful, AI is not intended to fully replace human experts. AI models rely on human expertise for quality control and to interpret complex results effectively. The most successful applications often involve a collaboration between humans and AI, where AI handles the initial analysis and pinpoints areas of interest, freeing experts to focus on nuanced interpretations and decision-making.
The future of cellular image analysis will depend on factors like the quality and quantity of training data available for AI, the standardization of imaging techniques, and advancements in making AI models more explainable.
5. Predicting cellular interactions
Cells within our bodies are like members of a bustling community, constantly communicating and influencing one another’s behavior. These cellular interactions form the foundation for everything from healthy growth to how diseases spread. Understanding this intricate cellular dialogue is like unlocking a biological treasure chest, offering new ways to combat disease and improve human health.
This is where Artificial Intelligence (AI) steps into the picture as a powerful detective. AI can analyze massive amounts of biological data, such as the detailed activity profiles of thousands of individual cells. Within this data lies a wealth of hidden patterns and relationships that might be too subtle for the human eye to catch. AI models can learn to recognize the molecular ‘handshakes’ that signify communication between cells, allowing them to predict new interactions that scientists may not have considered.
Moreover, AI’s power goes beyond identifying these interactions; it can also simulate what happens if a particular communication link is broken. This is akin to a virtual experiment, giving researchers insights into the potential effects of a drug before it’s even tested in the lab.
Different flavors of AI are used for this detective work. Some concentrate on analyzing patterns across vast collections of cell data, searching for pairs of cells whose activity seems consistently linked. Others take a more ‘spatially-aware’ approach, considering where the cells are located within a tissue since neighboring cells are more likely to be engaged in conversation.
Imagine the impact of AI-powered cellular interaction prediction – doctors could pinpoint exactly where communication goes wrong within a diseased tissue, opening the door to precisely targeted treatments. New drugs could be designed and tested much faster by simulating their impact on the entire cellular network. Eventually, we may see personalized medicine where doctors can predict a patient’s unique response to therapy based on their specific cellular makeup.
Which companies are at the forefront of AI applications in biology and bioengineering
At the forefront of AI-enabled cellular research is Colossal Biosciences, a Dallas-based company that has successfully reprogrammed Asian elephant cells to create stem cells for the first time in history.
These cells hold the potential to potentially bring back extinct mammoths, with scientists hoping to create a mammoth hybrid that looks exactly like its long-extinct counterpart. This breakthrough could pave the way for resurrecting woolly mammoths and help restore vulnerable Arctic tundras affected by global warming.
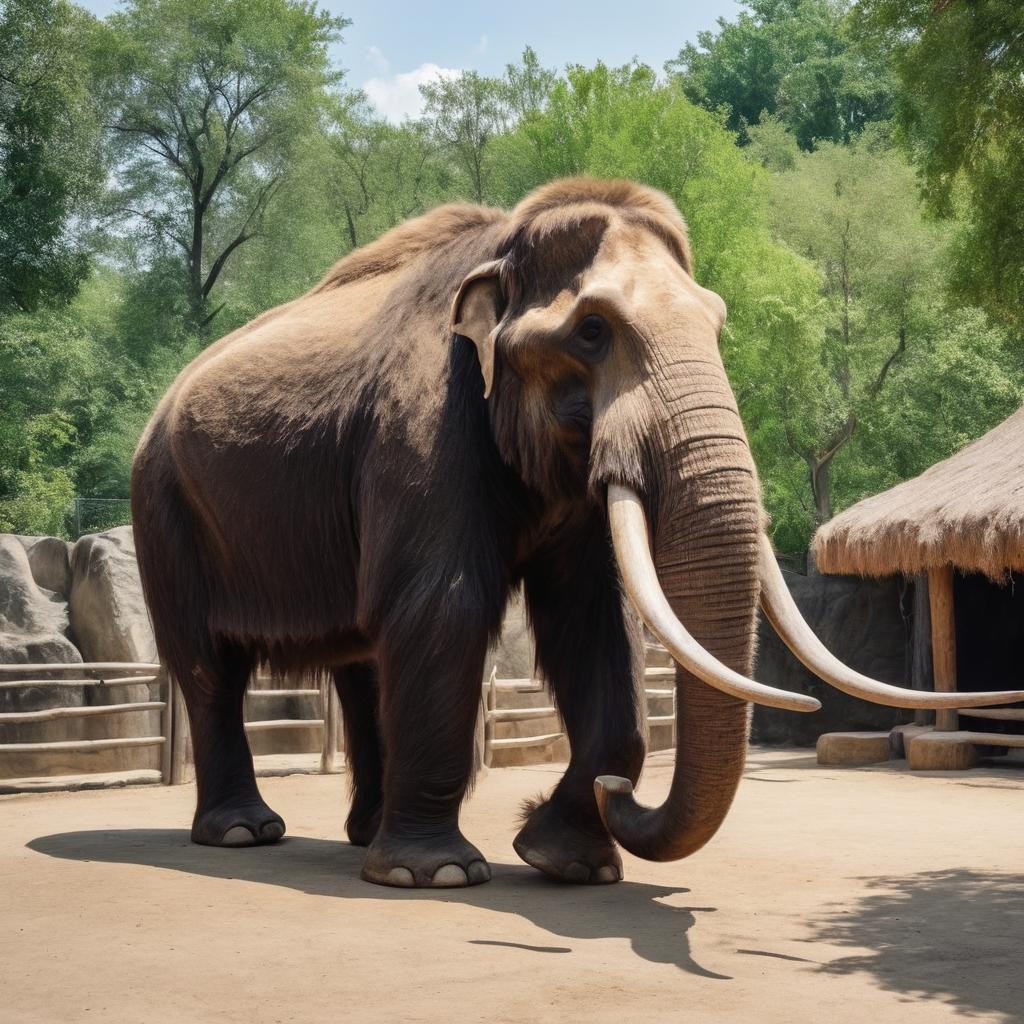
The creation of elephant stem cells and the potential resurrection of extinct mammoths through hybridization has the potential to revolutionize our understanding of conservation biology and impact human society in various ways. If successful, it could help restore vulnerable ecosystems like the Arctic tundra that are at risk due to global warming. This breakthrough is similar to the scenario depicted in the movie Jurassic Park as it involves bringing back extinct species using scientific methods.
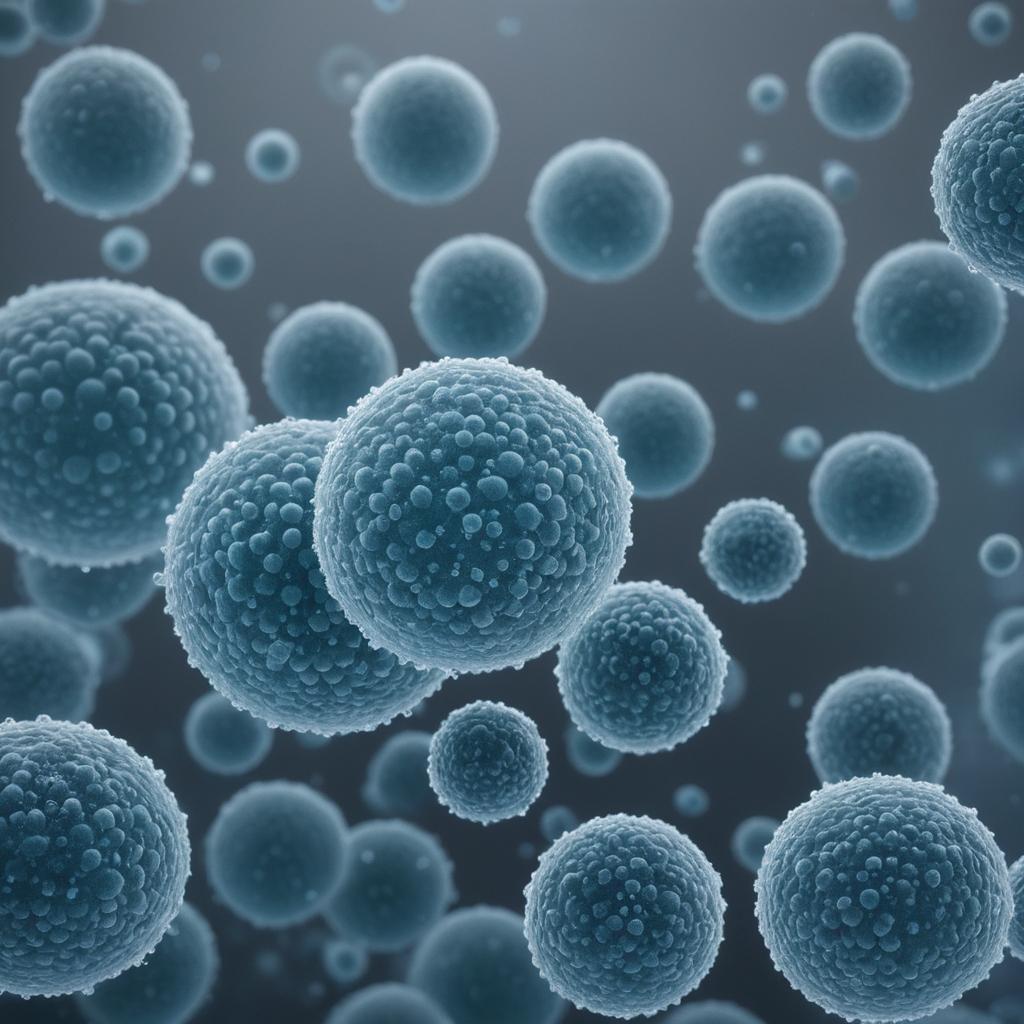
From micro-scale to cosmic scale
Another fascinating scientific discovery facilitated by AI models is the revelation of a mysterious galaxy that existed when the universe was only 700 million years old – the oldest “dead” galaxy ever observed. This revelation challenges the previously held belief that galaxies had formed through mergers, and instead suggests that they could have started as small objects before growing larger.
Furthermore, a loaf of bread discovered in Turkey has been identified as the world’s oldest known bread. Radiocarbon dating has revealed that the 5,000-year-old bread is much older than previously thought, shedding light on the evolution of bread and its significance throughout history.
Additionally, the discovery of toothless bird fossils in New Zealand challenges the widely held belief that birds evolved from dinosaurs with beaks. These new findings suggest that avian evolution may have been more complex than previously thought, with the possibility of multiple evolutionary paths for birds.
Moreover, evidence of ancient humans in Europe 1.4 million years ago has been discovered using a uniquely celestial method. The discovery of a star’s properties over time has enabled scientists to determine that ancient humans were present in Europe during this period.
Other notable discoveries include the revelation of tower pyramid-like star dunes that began forming thousands of years ago, a potential explanation for the fabled “death ray” of Archimedes through the lens of a 13-year-old boy’s insights, and differences in observing this year’s eclipse compared to the one that occurred in 2017.
As AI models become more powerful with the increased availability of cellular data, it is crucial to use them with caution as they may make mistakes due to their limitations. Nonetheless, these models hold immense potential for predicting what real cells would do in any situation and potentially creating new cells that don’t exist in nature.
Recent Comments